Artificial intelligence predicts malaria outbreaks in South Asia
Last reviewed: 14.06.2024
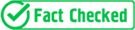
All iLive content is medically reviewed or fact checked to ensure as much factual accuracy as possible.
We have strict sourcing guidelines and only link to reputable media sites, academic research institutions and, whenever possible, medically peer reviewed studies. Note that the numbers in parentheses ([1], [2], etc.) are clickable links to these studies.
If you feel that any of our content is inaccurate, out-of-date, or otherwise questionable, please select it and press Ctrl + Enter.
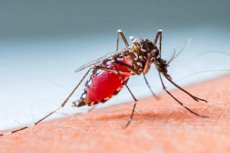
Researchers from NDORMS, in collaboration with international institutions, have demonstrated the potential of using environmental measurements and deep learning models to predict malaria outbreaks in South Asia. The study offers promising prospects for improving early warning systems for one of the world's deadliest diseases.
Malaria remains a significant global health problem, with the risk of infection affecting approximately half of the world's population, especially in Africa and South Asia. Although malaria is preventable, the variable nature of climate, sociodemographic and environmental risk factors makes predicting outbreaks difficult.
A team of researchers led by Associate Professor Sarah Khalid from the NDORMS Planetary Health Informatics Group, University of Oxford, in collaboration with Lahore University of Management Sciences, sought to solve this problem and explore whether an environment-based machine learning approach could offer potential for tools site-specific early warning for malaria.
They developed a multivariate LSTM model (M-LSTM) that simultaneously analyzed environmental indicators including temperature, rainfall, vegetation measurements and night-time light data to predict malaria incidence in the South Asian belt covering Pakistan, India and Bangladesh.
The data were compared to county-level malaria incidence rates for each country between 2000 and 2017, obtained from the US Agency for International Development's Demographic and Health Surveys datasets.
Results published in The Lancet Planetary Health show that the proposed M-LSTM model consistently outperforms the traditional LSTM model with errors of 94.5%, 99. 7% and 99.8% are lower for Pakistan, India and Bangladesh respectively.
Overall, higher accuracy and error reduction were achieved with increasing model complexity, highlighting the effectiveness of the approach.
Sarah explained: “This approach is universal and therefore our modeling has significant implications for public health policy. For example, it could be applied to other infectious diseases or scaled up to other high-risk areas with disproportionately high incidence and mortality from malaria in regions WHO in Africa This can help decision makers implement more proactive measures to manage malaria outbreaks early and accurately.
"The real attraction lies in the ability to analyze virtually anywhere on Earth thanks to rapid advances in Earth observation, deep learning and AI, and the availability of high-performance computers. This could lead to more targeted interventions and better allocation of resources in ongoing eradication efforts malaria and improve public health outcomes around the world."