AI-guided mammography reduces workload by 33% and increases breast cancer detection
Last reviewed: 14.06.2024
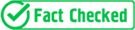
All iLive content is medically reviewed or fact checked to ensure as much factual accuracy as possible.
We have strict sourcing guidelines and only link to reputable media sites, academic research institutions and, whenever possible, medically peer reviewed studies. Note that the numbers in parentheses ([1], [2], etc.) are clickable links to these studies.
If you feel that any of our content is inaccurate, out-of-date, or otherwise questionable, please select it and press Ctrl + Enter.
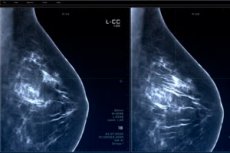
In a recent study published in Radiology, researchers from Denmark and the Netherlands conducted a retrospective analysis of screening effectiveness and the overall burden of mammography screening before and after the introduction of artificial intelligence (AI) systems.
Regular mammography screening for breast cancer significantly reduces mortality from the disease. However, mass mammography screening increases the workload of radiologists who must review many mammograms, most of which do not contain suspicious lesions.
In addition, double screening, which is used to reduce false positives and improve detection, further increases the workload of radiologists. The lack of specialized radiologists who can read mammograms exacerbates this situation.
Recent studies have extensively explored the use of AI to effectively analyze radiology reports while maintaining high screening standards. The combined approach, in which AI helps radiologists highlight mammograms with flagged lesions, is believed to reduce the workload of radiologists while maintaining screening sensitivity.
The current study used preliminary performance measures from two cohorts of women screened mammographically as part of the Danish National Breast Cancer Screening Program to compare the change in screening workload and performance after the introduction of AI tools.
The program invited women aged 50 to 69 years to be screened every two years until age 79. Women with markers indicating an increased risk of breast cancer, such as BRCA genes, were screened using different protocols.
The researchers used two cohorts of women: one screened before and one after the introduction of the AI system. Only women under 70 years of age were included in the analysis to exclude those in the high-risk subgroup.
All participants underwent standard protocols using digital mammographs with craniocaudal and mediolateral oblique views. All positive cases in this study were identified by screening for ductal carcinoma or invasive cancer, which were confirmed by needle biopsy. Data on pathological reports, lesion size, lymph node involvement and diagnoses were also obtained from the national health registry.
The AI system used to analyze mammograms was trained using deep learning models to detect, highlight and score any suspicious calcifications or lesions on a mammogram. The AI then ranked the screenings on a scale of 1 to 10, indicating the likelihood of breast cancer.
A team of mostly experienced radiologists reviewed mammograms for both cohorts. Before the implementation of the AI system, each screening was reviewed by two radiologists, and the patient was recommended for clinical examination and needle biopsy only if both radiologists considered the screening to require further evaluation.
After implementing the AI system, mammograms with a score less than or equal to 5 were reviewed by a senior radiologist, knowing that they only received one reading. Those that required further examination were discussed with a second radiologist.
The study found that the implementation of the AI system significantly reduced the workload of radiologists analyzing mammograms as part of mass breast cancer screening, while improving screening efficiency.
The cohort screened before the implementation of the AI system consisted of more than 60,000 women, while the cohort screened using AI was approximately 58,000 women. Screening with AI resulted in an increase in breast cancer diagnoses (0.70% pre-AI vs. 0.82% with AI) while reducing the number of false positives (2.39% vs. 1.63%).
AI-based screening had a higher positive predictive value, and the percentage of invasive cancers was lower with AI-based methods. Although the percentage of node-negative cancers did not change, other performance measures showed that AI-based screening significantly improved outcomes. The reading load also decreased by 33.5%.
The study therefore assessed the effectiveness of an AI-based screening system in reducing the workload of radiologists and improving screening rates of mammograms as part of mass breast cancer screening in Denmark.
The results showed that the AI-based system significantly reduced the workload of radiologists while improving screening rates, as evidenced by a significant increase in breast cancer diagnoses and a significant reduction in false positives.